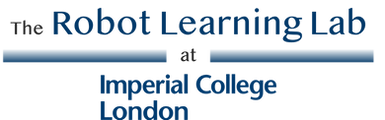
Team Members

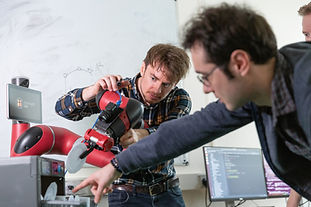
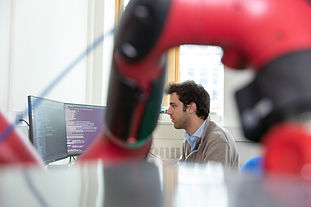
Edward Johns
Lab Director
​
​
​
​
​
​
​
​
​
​
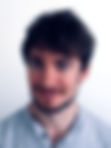
I am Director of the Robot Learning Lab at Imperial College London, where I am also a Senior Lecturer and a Royal Academy of Engineering (RAEng) Research Fellow. I received a BA and MEng in Electrical and Information Engineering from Cambridge University, and a PhD in vision-based robot localisation from Imperial College, supervised by Guang-Zhong Yang in the Hamlyn Centre. Following my PhD, I spent a year as a postdoc at UCL working with Gabriel Brostow, and I then returned to Imperial College as a founding member of the Dyson Robotics Lab with Andrew Davison, where I led the robot manipulation team. In 2017, I was awarded an RAEng Research Fellowship, and then in 2018 I was appointed as a Lecturer at Imperial College, and founded the Robot Learning Lab. Alongside leading the lab's research, I teach a graduate-level course on Robot Learning.
Kamil Dreczkowski
PhD student (started 2019)
​
​
​
​
​
​
​
​
​
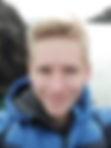
Before beginning my PhD, I completed a Bachelor’s degree in Physics and a Master’s degree in Computing (Machine Learning) at Imperial College London. During my Master’s degree I completed my individual project under the supervision of Dr. Edward Johns. The focus of this project was on learning stochastic policies from demonstrations and a sparse reward function. My PhD will extend this project and will investigate methods for robot learning of contact-rich task from demonstrations and a sparse reward function, which are both safe and efficient, and are suitable for deployment in an industrial setting.
​
Publications
IROS 2024 Adapting Skills to Novel Grasps: A Self-Supervised Approach
CoRL 2023 One-Shot Imitation Learning: A Pose Estimation Perspective
CoRL 2021 Learning Eye-in-Hand Calibration from a Single Image
IROS 2021 Hybrid ICP
Norman Di Palo
PhD student (started 2020)
​
​
​
​
​
​
​
​
​
​

I received a BSc. in Control Engineering from University of Naples Federico II and an MSc. in AI & Robotics from Sapienza University of Rome. Over the years, I had the opportunity to work and conduct research at several institutes, startups and universities around the world. In the summer of 2017 I visited Tohoku University (Sendai, Japan), working on wheeled robots for space exploration. In the summer of 2018 I’ve been an AI research intern at Curious AI (Helsinki, Finland), where I developed state-of-the-art techniques in model-based reinforcement learning. In the summer of 2019 I conducted research at the Italian Institute of Technology (Genoa, Italy), working on quadruped robots. I joined the Robot Learning Lab first as a visiting researcher during the winter of 2019, and was then accepted for a PhD the following year. I research and design methods that allow robots and humans to collaborate in novel, intuitive and effective ways.
​
Publications
ICRA 2025 R+X: Retrieval and Execution from Everyday Human Videos
RSS 2024 Keypoint Action Tokens Enable In-Context Imitation Learning in Robotics
RA-L 2024 Language Models as Zero-Shot Trajectory Generators
ICRA 2024 DINOBot: Robot Manipulation via Retrieval and Alignment with Vision Foundation Models
RA-L 2024 On the Effectiveness of Retrieval, Alignment, and Replay in Manipulation
CoRL 2021 Learning Multi-Stage Tasks with One Demonstration via Self-Replay
IROS 2021 Coarse-to-Fine for Sim-to-Real: Sub-Millimetre Precision Across Wide Task Spaces
Vitalis Vosylius
PhD student (started 2020)
​
​
​
​
​
​
​
​
​

I am a PhD student at Imperial College London, working in the Robot Learning Lab. Prior to joining Imperial College, I received a Bachelor’s degree in Applied Physics from Vilnius University, Lithuania. Following my studies, I also carried out research with a focus on laser beam shaping. Before becoming a PhD student, I completed an MSc in Artificial Intelligence at Imperial College London. I completed my MSc individual project under the supervision of Dr. Edward Johns. During the project, I investigated ways of using Deep Learning to increase the efficiency of planning algorithms, focusing on robot motion planning. My current research interests are robot manipulation, hierarchical planning and efficient replanning for completing complex tasks.
​
Publications
ICLR 2025 Instant Policy: In-Context Imitation Learning via Graph Diffusion
IROS 2024 Adapting Skills to Novel Grasps: A Self-Supervised Approach
CoRL 2023 Few-Shot In-Context Imitation Learning via Implicit Graph Alignment
RA-L 2023 DALL-E-Bot: Introducing Web-Scale Diffusion Models to Robotics
CoRL 2022 Where to Start? Transferring Simple Skills to Complex Environments
Ivan Kapelyukh
PhD student (started 2021)
​
​
​
​
​
​
​
​
​
​

I am a PhD student at Imperial College London jointly supervised by Dr. Edward Johns at the Robot Learning Lab and Prof. Andrew Davison at the Dyson Robotics Lab. Before joining the team, I graduated with First Class Honours from the four-year Computing MEng programme at Imperial College. My MEng thesis was supervised by Dr. Edward Johns. It focused on learning tidying preferences using techniques such as graph neural networks, variational autoencoders and word embeddings from natural language processing. My research is centred around aligning robot goals with human interests. This includes ideas from Inverse Reinforcement Learning and Computer Vision, as well as novel methods which learn with minimal human supervision.
​
Publications
ICRA 2024 Dream2Real: Zero-Shot 3D Object Rearrangement with Vision-Language Models
RA-L 2023 DALL-E-Bot: Introducing Web-Scale Diffusion Models to Robotics
CoRL 2021 My House My Rules: Learning Tidying Preferences with Graph Neural Networks
Georgios Papagiannis
PhD student (started 2021)
​
​
​
​
​
​
​
​
​
​
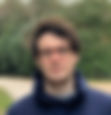
Before joining the Robot Learning lab, I obtained a BSc. in Computer Science from the University of Surrey in 2020, where I worked on improving the performance of adversarial Imitation Learning (IL) methods by formulating IL as minimisation of the Sinkhorn distance between the demonstrator’s and learner’s trajectories. Following my BSc., I obtained an MPhil in Advanced Computer Science from the University of Cambridge. As an MPhil student I spent the majority of my time conducting research which focused on developing an automatic curriculum learning method for Reinforcement learning agents. Currently for my PhD, my research is focused on developing new methods to teach robots to perform contact-rich manipulation tasks in the real-world efficiently from human demonstrations.
​
Publications
ICRA 2025 R+X: Retrieval and Execution from Everyday Human Videos
CoRL 2024 MILES: Making Imitation Learning Easy with Self-Supervision
​IROS 2024 Adapting Skills to Novel Grasps: A Self-Supervised Approach
IROS 2022 Demonstrate Once, Imitate Immediately: Learning Visual Servoing for One-Shot Imitation Learning
Pietro Vitiello
PhD student (started 2022)
​
​
​
​
​
​
​
​
​
​

I am an Italian currently pursuing a PhD in the Robot Learning Lab at Imperial College London. In 2021 I graduated from Imperial College with an MEng in Biomedical Engineering, during which I worked on several projects which opened my eyes to the fascinating topic of machine learning and computer vision. To strengthen my foundations I subsequently decided to take an MSc in Artificial Intelligence and Machine Learning at Imperial College, from which I graduated in 2022. In the last months of this Masters degree I had the pleasure to research towards finding an alternative visual representation for robot actions in visual robot learning. Currently, my interests lie at the intersection of Robotics and Computer Vision and are focused on few-shot Imitation Learning for robot manipulation. Ultimately, I aim at developing methods through which a robot can learn everyday object interaction skills, while minimising the required amount of human supervision.
​
Publications
ICRA 2025 R+X: Retrieval and Execution from Everyday Human Videos
CoRL 2023 One-Shot Imitation Learning: A Pose Estimation Perspective
Yifei Ren
PhD student (started 2022)
​
​
​
​
​
​
​
​
​
​
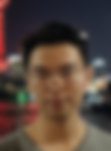
I'm a PhD student at Imperial College London, working in the Robot Learning Lab supervised by Dr. Edward Johns. I received my BEng degree in Intelligent Science and Technology from Nankai University in China, where I worked on Robotics and Computer Vision. After that, I completed my MSc with Distinction at Imperial College London, during which my main focus was on object-level SLAM system. Currently, my interests lie in Robotics, Computer Vision and SLAM, and my current research is focussed on the intersection of 3D Computer Vision and Robot Manipulation, such as bringing 3D information to Imitation Learning frameworks to enable high accuracy
​
Publications
ICRA 2024 Dream2Real: Zero-Shot 3D Object Rearrangement with Vision-Language Models
Naoki Kiyohara
PhD student (started 2023)
​
​
​
​
​
​
​
​
​
​
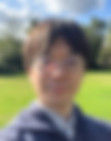
I am from Tokyo, Japan. I graduated with a bachelor's degree in Physics at Tokyo University of Science and a master’s degree in Advanced Materials Science at the University of Tokyo. Subsequently, I joined Canon Inc. (Tokyo) and am now at Imperial College London as a PhD student, sponsored through the company's corporate study abroad program. My research is centered around model-based reinforcement learning, with a particular focus on sequential generative models. Moving forward, I aim to significantly enhance exploration efficiency by utilizing models that can capture uncertainties, such as Bayesian models. In my free time, I enjoy playing and listening to classical piano music. I am co-supervised by Edward Johns and Yingzhen Li.
Yilong Wang
PhD student (started 2024)
​
​
​
​
​
​
​
​
​
​

I completed an MEng in Electronic and Information Engineering at Imperial College London, where my thesis research at the Personal Robotics Lab, supervised by Professor Yiannis Demiris, focused on mobile manipulation for assistive cooking. Following this, I pursued an MRes in Artificial Intelligence and Machine Learning, concentrating on sample-efficient dual-arm imitation learning under Dr. Edward Johns. Currently, in my PhD research, I am developing new dual-arm manipulation techniques aimed at approaching human-level proficiency.
​​
Publications
ICRA 2025 One-Shot Dual-Arm Imitation Learning
Ruomeng Fan
PhD student (started 2024)
​
​
​
​
​
​
​
​
​
​

Hello! I began my Ph.D. journey in October 2024, supervised by Dr. Edward Johns. Previously, I completed my BSc in Automation at Beijing Institute of Technology. To broaden my horizons, I worked on several robotics topics such as locomotion, manipulation and teleoperation. Following that, I went to the University of Tokyo for an MEng in Mechanical Engineering under the supervision of Professor Yuji Yamakawa. My research there was about robot manipulation learning from single human observation — a direction that really shaped my interest in efficient robot learning. Then I spent one year working as a project researcher in the same lab and travelling around Japan. I joined the Robot Learning Group at Imperial with the motivation of exploring how to leverage data to enable robots in versatile tasks and to develop general purpose real-world robotics.
Cheng Qian
PhD student (started 2025)
​
​
​
​
​
​
​
​
​
​

Before starting my PhD at the Robot Learning Lab at Imperial College London, I completed my BSc and MSc in Electrical Engineering and Information Technology at the Technical University of Munich. I conducted my master's thesis research at the Technical University of Darmstadt under the supervision of Prof. Jan Peters, where I explored teaching bimanual dexterous robot hands to perform piano pieces like human pianists. For my PhD, my primary research interest lies in developing efficient imitation learning methods for versatile and precise robotic manipulation tasks, particularly by leveraging human demonstrations.